The vision for the future of warfare is clear: “robots, not humans, should fight on the battlefield”, as stated by Ukraine’s deputy minister of digital transformation Alex Bornyakov.1 Many ambitious and techno-optimist defence tech companies across Ukraine, Europe, and the U.S., committed to innovation on the battlefield to protect democracies and reduce human suffering, are advancing AI with the goal of removing warfighters from direct combat and replacing them with autonomous unmanned systems. After three years of integrating cutting-edge AI-driven technology into combat, Ukraine and its allies have learned hard lessons about the complexities of human-machine collaboration in high intensity warfare. AI-enabled, attritable autonomous, and swarm-enabled systems are key to overcoming numerical disadvantages. Yet, the reality in early 2025 is that, while AI plays an indispensable role in military operations, full autonomy —- a robot performing all aspects of a task autonomously without human intervention in adherence with established tactics, procedures and operational concepts, while also being capable of coordinated and independent actions and decisions2 — is not yet present on the battlefield.3 Nonetheless, AI has proven indispensable for critical functions, such as navigation and targeting in GNSS-denied environments. Mykhailo Fedorov, Ukraine’s deputy prime minister and technology chief, acknowledges that AI-powered systems do not yet make independent decisions to strike, though he believes this is the direction of future development.4 Meanwhile, sceptics like Jim Acuna, a former CIA officer, argue that true battlefield autonomy is wishful thinking.5
The question of autonomy is more nuanced with various levels — as outlined by NIST’s Autonomy Levels for Unmanned Systems Framework ranging from full human control (0) to fully autonomous operations with no human involvement (5). 6 We are progressively advancing toward higher levels of mission autonomy. Emerging technologies are enabling novel functions in Manned-Unmanned Teaming, such as swarming. In this scenario, minimal human involvement is present in the coordinated deployment of multiple (semi-)heterogenous uncrewed platforms, payloads and systems that exercise collaborative autonomy in performing tasks autonomously and making real-time tactical decisions inside the decentralised network.7 This is not a distant future, there are several defence companies competing in developing or deploying such software to enable a single operator to interface various autonomous assets simultaneously. This addresses operational efficiency in high intensity warfare and might also ease the ongoing recruitment challenges faced by military forces in Europe and United States.8
War has always been a collaboration between humans and machines. However, the relationship between humans and machines is becoming increasingly interdependent as they work together to navigate the growing complexity and fast-changing dynamics of modern battlefields. While the balance between humans and machines in warfare may shift in the future as operations demand more system autonomy, it is crucial to understand how they collaborate effectively. This requires examining factors such as hardware, software, and human elements that shape their interaction.
Human-Machine Teaming (HMT) is a “complex military process with a feedback loop between the human and the machine which changes the behaviour of both.” Central to this approach is the collaborative execution of battlefield tasks, where humans and AI leverage their respective strengths. Humans contribute contextual thinking, intuition from warfighting experience, and creativity, while AI excels in processing vast amounts of data, maintaining precision, speed, and consistency without fatigue or attention limitations.9
This article explores the evolving concept of human-machine teaming (HMT) in modern warfare and how it enhances operational efficiency. It examines the latest AI advancements enabling HMT, including object detection through YOLO algorithms and AI-assisted air combat systems designed to reduce pilot’s cognitive load. Two major defence programs — FCAS in Europe and Project Maven in the United States — are highlighted to demonstrate how governments and the private sector are actively working to bring AI technological advancements into operational capabilities.
1. Latest technological developments enabling HMT
As warfare becomes increasingly software-centric, the advantage lies in software's adaptability over hardware systems, to learn and adapt through rapid iteration cycles enabling swift updates based on new data streams or past battlefield operational insights. There are two main pathways for technological development. First, commercial applications often evolve into dual-use functions, finding applications in both civilian and military domains. Second, existing defence software improves over time due to the nature of machine learning, whether through supervised or unsupervised learning.
Reinforcement learning, and deep neural networks, allow these algorithms to analyse vast amounts of historical and real-time multi-modal data streams. By recognizing patterns in troop movements, enemy tactics, and sensor inputs, they make more accurate predictions and operational recommendations, enabling their system to learn from trial and error, refining their decision-making over time. In these fast-learning cycles, human oversight remains a key factor in improving battlefield AI. AlphaGo’s Move 37, an unconventional yet highly effective play, showcased AI’s potential for unpredictable brilliance in decision-making. Similarly, AI-driven military decision-support systems could propose counterintuitive courses of action, driving commanders to weigh AI recommendations against traditional strategic wisdom.10 Human-in-the-loop (HITL) systems ensure that soldiers and commanders review AI-generated insights, correcting errors and refining the system’s outputs to enhance situational awareness, targeting accuracy, logistics, and overall decision-making in combat environments. Given the complexity of battlefield tasks, AI-driven capabilities can assist in various functions throughout the Observe-Orient-Decide-Act (OODA) loop, including two exciting ongoing developments — AI-enabled object detection and AI copilots at edge— some of which have already been deployed in Ukraine.
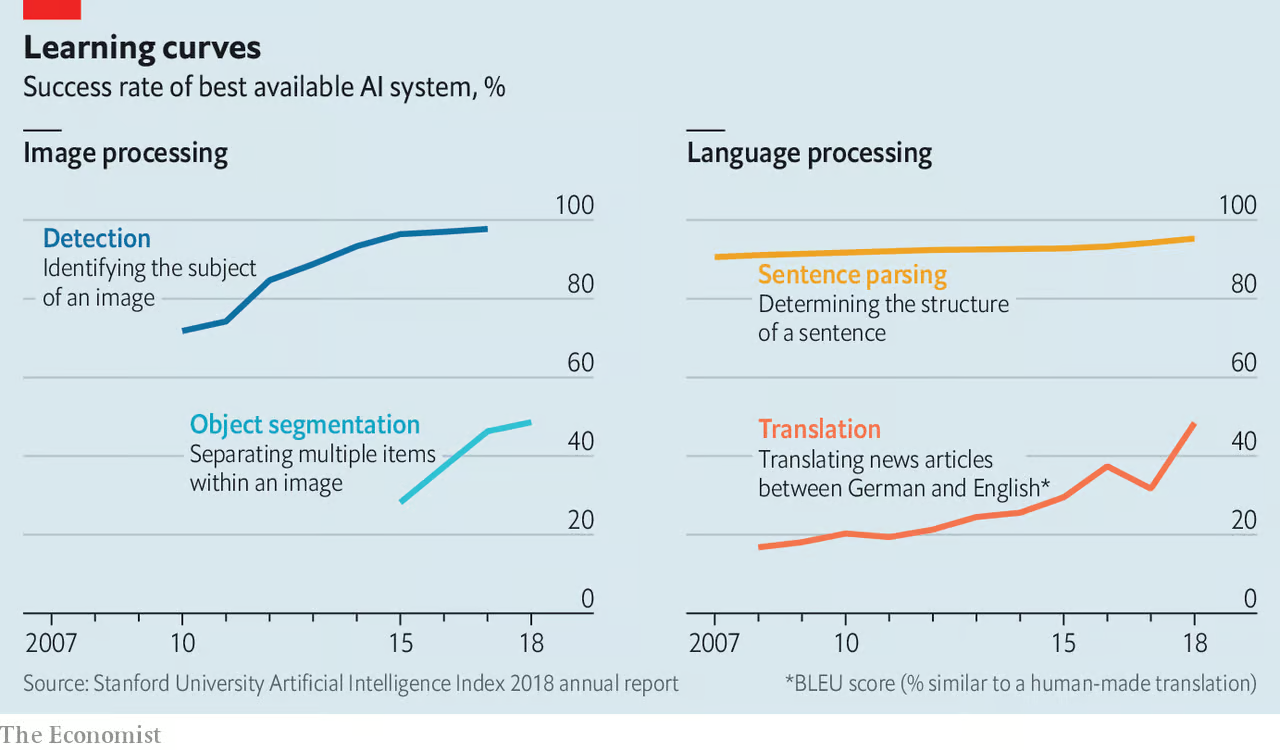
1.1 YOLO object detection algorithm
When unmanned autonomous vehicles are used for ISR (intelligence, surveillance, and reconnaissance) missions, human-machine teaming is structured so that humans concentrate on the final stages of decision-making and authorizing actions, while AI algorithms aid in detecting, localizing, and classifying objects on the battlefield. This collaboration is crucial for accelerating decision-making in response to diverse threats across land, air, and maritime domains, with AI closing the OODA loop to help military personnel swiftly analyse and act in dynamic battlefield situations.
Object detection is an important task in computer vision, dealing with localizing a region of interest within an image and classifying this region like a typical image classifier.12 YOLO (You Only Look Once) is a popular object detection model based on convolutional neural networks and was first introduced by Joseph Redmon et al. in 201613 and has since undergone several iterations, the latest being YOLO12 with key features of area attention mechanism, Residual Efficient Layer Aggregation Networks (R-ELAN) and flexible deployment, improving various tasks of detection, segmentation, pose, classification, the output labelled Oriented Bounding Boxes (OBB).14
YOLO is increasingly adopted in defence applications as it outperforms traditional detection methods like radar and optical systems by providing faster and more accurate identification of military aircraft, drones, and other threats, even in challenging conditions, mitigating low visibility areas.15 YOLO’s lightweight design allows it to run on commercial hardware and be easily integrated into existing military systems, offering a cost-effective solution while reducing human error.16
YOLO is a one-stage object detection model — in contrast with the previously widely used two stage RCNN model — thus uses a single pass of the input image to predict the presence and location of the objects in the image, which is crucial for localizing and classifying military objects in real time.17 By processing an entire image in a single pass, these algorithms offer high computational efficiency, making them ideal for resource-constrained environments. While they may be less accurate than other methods, especially for small objects, their speed and efficiency enhance situational awareness in military operations.
The development of object detection algorithms is part of a bigger challenge of deploying them. Deployment issues that can negatively impact YOLO model performance include, integration of models for edge devices with limited compute resources, running models in unforeseen scenarios making offline retraining unfeasible. Therefore, current research focuses on in-mission learning, addressing challenges like camouflaged objects, unknown variants, object detection techniques for other than RGB images inputs.18
YOLO’s real-time object detection capabilities significantly enhance human-machine collaboration in defence applications by swiftly identifying military threats, such as missile launchers and aircraft, and classifying friend or foe objects. YOLO allows soldiers to focus on critical decision-making, while the system handles (near) real-time detection and classification tasks autonomously. This collaboration improves operational efficiency, enabling quicker responses in high-stakes situations overcoming manual labelling in high intensity scenarios.
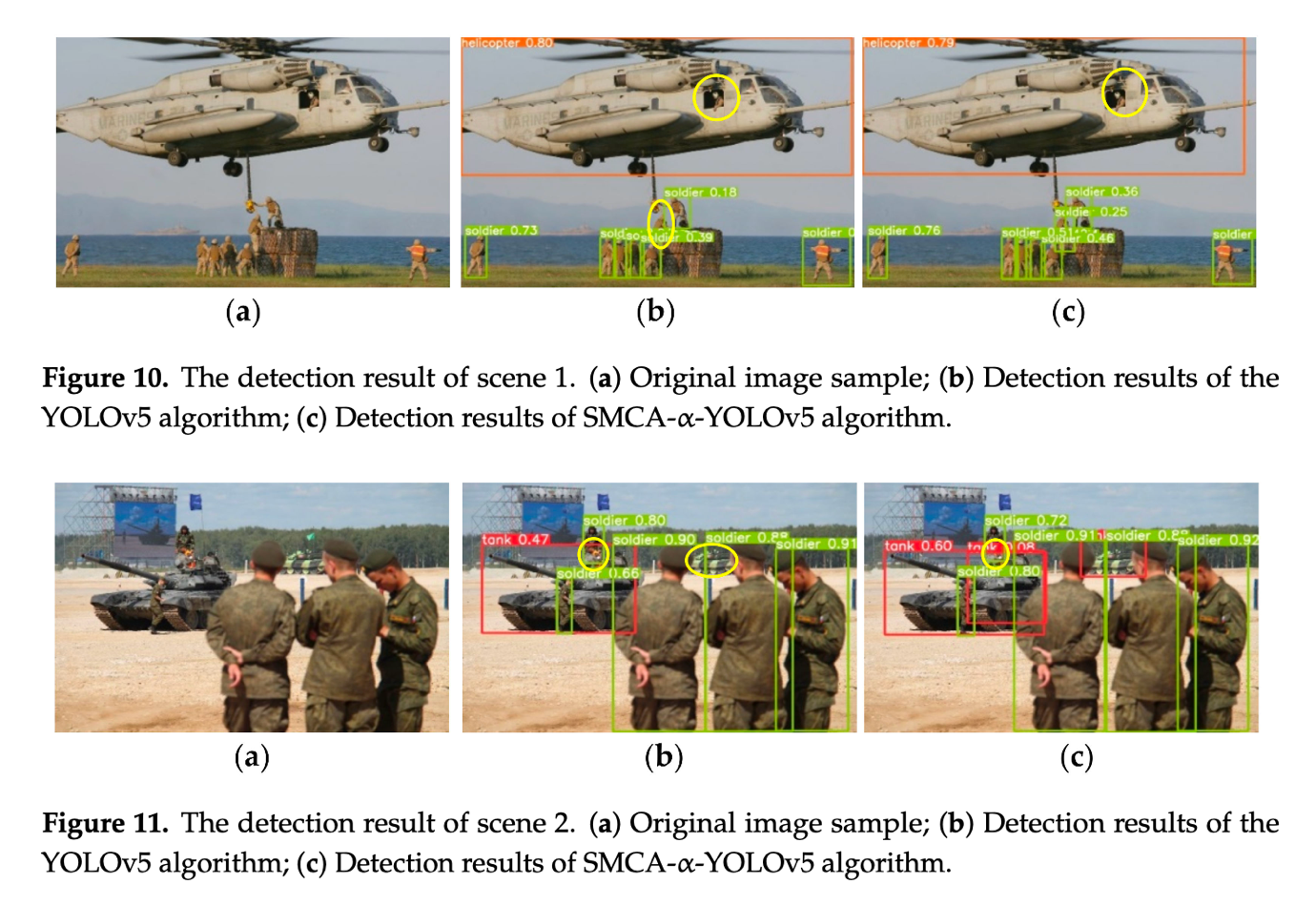
1.2 Project Maven: object detection algorithms to enhance HMT in ISR missions inside the Pentagon
As militaries increasingly rely on artificial intelligence to enhance battlefield decision-making, the U.S. Department of Defense has heavily invested in AI-enabled object detection through Project Maven. Established in 2017, Project Maven was designed to integrate advanced machine learning algorithms into military intelligence, surveillance, and reconnaissance (ISR) operations.20 Originally developed to automate the processing of vast amounts of reconnaissance data, it has since transitioned to the National Geospatial-Intelligence Agency (NGA) in 2023, becoming a Program of Record and a core component of the Pentagon’s broader Combined Joint All-Domain Command and Control (CJADC2) initiative.21
YOLO algorithm is one such model that is designed to identify and classify military-relevant objects such as tanks, radars, and missile launchers from ISR footage. This automation significantly reduces the burden on human analysts, who would otherwise spend hours manually reviewing full-motion video (FMV) from surveillance drones. 22 By compressing the traditional "kill chain", Project Maven accelerates the OODA loop, allowing military units to rapidly process intelligence and act on it.
A central aspect of Project Maven’s AI integration is its role in Human-Machine Teaming. While algorithms can swiftly identify objects and detect patterns across optical, thermal, radar, and synthetic aperture radar imagery, human operators remain in control of targeting decisions. This ensures that while AI speeds up intelligence processing and battlefield awareness, critical engagements are still governed by human judgment. The Maven Smart System further enhances this synergy by linking operators, sensor feeds, and AI-driven analytics into a unified interface, improving both speed and accuracy in tactical decision-making.23
By automating surveillance and object recognition, Project Maven aims to alleviate the cognitive workload on human personnel while increasing the efficiency of military operations. U.S. Air Force ISR leadership has warned of the risk of "drowning in data" due to the overwhelming volume of surveillance footage generated by drones.24 Project Maven helps mitigate this challenge by enabling AI-assisted analysis, potentially doubling or tripling analyst productivity while reducing the personnel required for targeting operations from 2,000 to just 20.25 However, despite these advances, AI still struggles with certain identification challenges, such as differentiating vehicles from natural objects in complex environments like deserts, snow-covered terrains, or urban settings where decoys may be deployed.26 Additionally, AI remains limited in determining optimal attack sequences and weapon selection,27 and underperforms compared to humans in domain adaptability, in other words being able to apply knowledge learned in one context to another, therefore these are tasks that still require human expertise.28
Ultimately, Project Maven represents a significant step toward integrating AI-powered object detection into modern warfare. By fusing vast amounts of sensor data with machine learning and human oversight, it enhances situational awareness, speeds up military decision-making, and exemplifies the evolving role of human-machine teaming on the battlefield. However, its limitations underscore the continuing need for human expertise in critical engagement decisions.
2. HMT for Collaborative Air Dominance
2.1 AI Copilots
AI copilots are emerging as a critical function in the air domain, offering support to human pilots by enhancing their abilities, intuition, and decision-making. Rather than seeking to replace pilots, the goal is to augment their performance by leveraging machine speed and precision in situational awareness in various tasks such as mission planning, command and control, or for training purposes. 29 These AI copilots can either operate in the background, maintaining situational awareness, tracking blind spots, and alerting the pilot when needed, or step forward to take on the role of the pilot, executing evasive manoeuvres or flying the aircraft to protect the human pilot in critical situations.30
In various aviation hardware, including fighter jets and drones, AI serves as the backbone, supporting everything from flight navigation to complex mission execution. One remarkable example of AI's potential in aviation is ALPHA, an AI system that has repeatedly defeated human pilots in flight simulator trials. 31ALPHA’s ability to process vast amounts of sensor data and make rapid, accurate decisions far outpaces human capabilities. While human pilots have an average visual reaction time of 0.15 to 0.30 seconds, AI systems like ALPHA can process information and respond in a fraction of that time, giving them a distinct advantage in high-stakes, dynamic combat scenarios. This rapid decision-making ability, coupled with its capacity to run on inexpensive computing platforms, makes AI copilots not just a feasible but also a cost-effective solution for modern warfare.
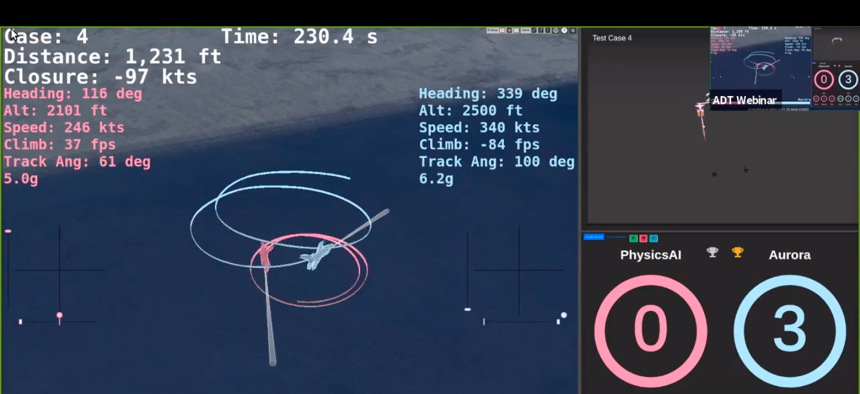
Johns Hopkins APL scientists and engineers are working on developing ML techniques for their VIPR (Virtual Intelligent Peer-Reasoning) agent, a copilot for situational awareness, performance and cognitive support. 33 VIPR leverage advanced machine learning models to enhance human-machine teaming. Key models include Recurrent Conditional Variational Autoencoders (RCVAEs), which enable VIPR to understand a pilot’s cognitive state and intentions; Graph Neural Networks (GNNs), used to predict adversary behaviour with high precision; and State-Time Attention Networks (STAN), which allow VIPR to adapt to dynamic environments and manage multiple tasks simultaneously.
Reinforcement learning-based AI plays a central role in developing these advanced copilots.34 By simulating trial-and-error processes, AI systems learn to interpret their environments, make decisions, and adapt strategies, mimicking human decision-making but with superior speed and accuracy. In combat situations, where quick thinking and precise actions are critical, AI copilots can reason over highly dynamic scenarios, such as predicting enemy movements and determining optimal positions for weapon effectiveness and pilot safety. This ability to process real-time data from multiple sources and make split-second decisions is invaluable in complex warfare environments.
The application of AI copilots goes beyond traditional simulation-based systems. For example, Shield AI’s Hivemind, a leading player in AI pilot autonomous systems, has adapted its AI by splitting the system into modular blocks, enabling the technology to be reconfigured for various missions in the real world.35 This adaptability opens up new possibilities for using AI in different combat situations, allowing for the effective deployment of autonomous systems in GPS and GNSS-denied environments, such as those seen in Ukraine, where electronic warfare disruptions are common.
One of the key benefits of AI copilots is their ability to reduce cognitive load on human pilots. By offloading routine tasks, monitoring systems, and even executing certain manoeuvres autonomously, AI copilots allow pilots to focus on higher-level strategic decisions.36 This collaboration not only enhances pilot performance but also ensures the survivability of manned assets in challenging operational conditions. Furthermore, AI copilots can employ tactics that humans may not have considered, offering surprising strategies that can be used to train future pilots, pushing the boundaries of human creativity and combat effectiveness.
The integration of AI copilots into military operations is further supported by simulations and war games, where AI models are tested in virtual environments that replicate a wide range of combat scenarios.37 These simulated settings, often using synthetic data when real battlefield data is limited, help prepare AI systems for unpredictable situations, ensuring they can effectively support human pilots when deployed in real-world operations.38 By combining the strengths of AI with human judgment and intuition, AI copilots represent a significant leap forward in human-machine teaming, promising to enhance both the speed and accuracy of decision-making and human performance in beyond-visual-range (BVR) air combat.
2.2 FCAS: Human-Machine Teaming in air combat for Europe
The Future Combat Air System (FCAS) is Europe’s flagship defence initiative, integrating AI copilots and autonomous systems to revolutionize air combat, alleviate the cognitive load to pilots and establish air superiority.39 As a joint project involving Germany, France, and Spain set for operational deployment by 2040, FCAS will feature AI as a central component that require advanced Human-Machine Teaming integrating human pilots, AI copilots, autonomous drones, and edge computing.
AI Copilots and the Evolution of the Pilot's Role
FCAS redefines the role of pilots, shifting them from direct control to mission operators.40 AI copilots will handle flight management and operational decisions, allowing pilots to focus on strategy and coordination. Unmanned aircraft will have the option to fly autonomously, demonstrating formation flying, tactical manoeuvring, and risk assessment, ensuring mission effectiveness without human oversight but with maintaining pilot awareness for trust and safety. Unlike conventional automation, FCAS AI supports real-time decision-making without requiring manual commands.
Loyal Wingmen and Decentralized Autonomy
Helsing’s AI Backbone ensures standardized, interoperable AI workflows for FCAS. 41 Part of Germany’s "National Research and Technology (R&T) Project NGWS," this initiative provides a centralized, secure platform for AI development, overcoming fragmented processes and accelerating mission planning, sensor data evaluation, and combat decision-making. FCAS employs onboard AI through edge computing, eliminating reliance on ground stations. AI systems will operate directly on the aircraft processing data in real time for rapid decision-making in dynamic combat scenarios.
A key feature of FCAS is its "loyal wingmen"—autonomous drones that enhance missions through intelligence gathering, added firepower, and enemy saturation.42 These drones use localized AI models for independent operation, continuously exchanging data through secure optical, radio, and infrared channels to maintain connectivity. Manned-Unmanned Teaming (MUM-T) is central to FCAS, with drones acting as remote carriers for intelligence, surveillance, target acquisition, and reconnaissance (ISTAR) missions. These platforms extend operational reach, supporting rescue operations, maritime surveillance, and border security.
Conclusion: status quo and where next with HMT?
The integration of AI into modern warfare is not a distant future but an evolving reality, reshaping the battlefield through human-machine teaming. AI-driven technologies such as object detection, AI copilots, and swarm-enabled systems are already enhancing operational efficiency, decision-making, and situational awareness delegating more and more mission autonomy to machines. As we deploy and operationalise rapid technological advancements, human oversight remains crucial to ensuring ethical, strategic, and adaptive use of AI in combat, leveraging human strengths like intuition and contextual understanding.
As autonomy on the battlefield continues to grow, defence technology companies have been actively testing their systems in Ukraine since 2022, with many establishing local offices to trial their latest innovations in real-world combat environments. 43 NATO is also leveraging these insights, setting up the NATO-Ukrainian Joint Analysis Training and Education Centre (JATEC) in Poland to incorporate operational lessons from the war in Ukraine into its defence planning and warfighting concepts.44 Ultimately, fostering a collaborative relationship between AI and human decision-makers will be key to navigating the complexities of high-intensity conflicts while maintaining ethical and strategic oversight.
References
9 https://static.rusi.org/human-machine-teaming-sr-jan-2024.pdf
10 https://ora.ox.ac.uk/objects/uuid:4ccbb114-71f9-4e3a-99ea-f8b3232e8bc1
12 https://www.v7labs.com/blog/yolo-object-detection
14 https://docs.ultralytics.com/models/yolo12/#key-improvements
15 https://journals.chnu.edu.ua/sisiot/article/view/568
16 https://www.nature.com/articles/s41598-025-85488-z
17 https://www.v7labs.com/blog/yolo-object-detection#single-shot-object-detection
19 https://www.mdpi.com/2079-9292/11/20/3263
22 https://link.springer.com/chapter/10.1007/978-3-030-73276-9_10
24 https://geographicalimaginations.com/2015/06/10/digital-capture-and-physical-kill/
26 https://www.rand.org/content/dam/rand/pubs/research_reports/RRA800/RRA866-1/RAND_RRA866-1.pdf
27 https://defensetalks.com/united-states-project-maven-and-the-rise-of-ai-assisted-warfare/
29 https://shield.ai/hivemind-for-operational-read-and-react-swarming/
30 https://www.jhuapl.edu/news/news-releases/240618-ai-copilot-future-air-combat
31 https://www.wired.com/2016/06/ai-fighter-pilot-beats-human-no-need-panic-really/
32 https://www.defenseone.com/technology/2020/08/ai-just-beat-human-f-16-pilot-dogfight-again/167872/
33 https://www.jhuapl.edu/news/news-releases/240618-ai-copilot-future-air-combat
35 https://shield.ai/hivemind-for-operational-read-and-react-swarming/
37 https://www.defensenews.com/air/2024/04/19/us-air-force-stages-dogfights-with-ai-flown-fighter-jet/
38 https://defensescoop.com/2024/04/12/ai-wargaming-air-force-futures-mit/
39 https://www.airbus.com/en/products-services/defence/future-combat-air-system-fcas
41 https://helsing.ai/newsroom/ai-backbone-for-fcas-operational
42 https://www.eurasiantimes.com/bodyguards-of-future-fighter-jets/